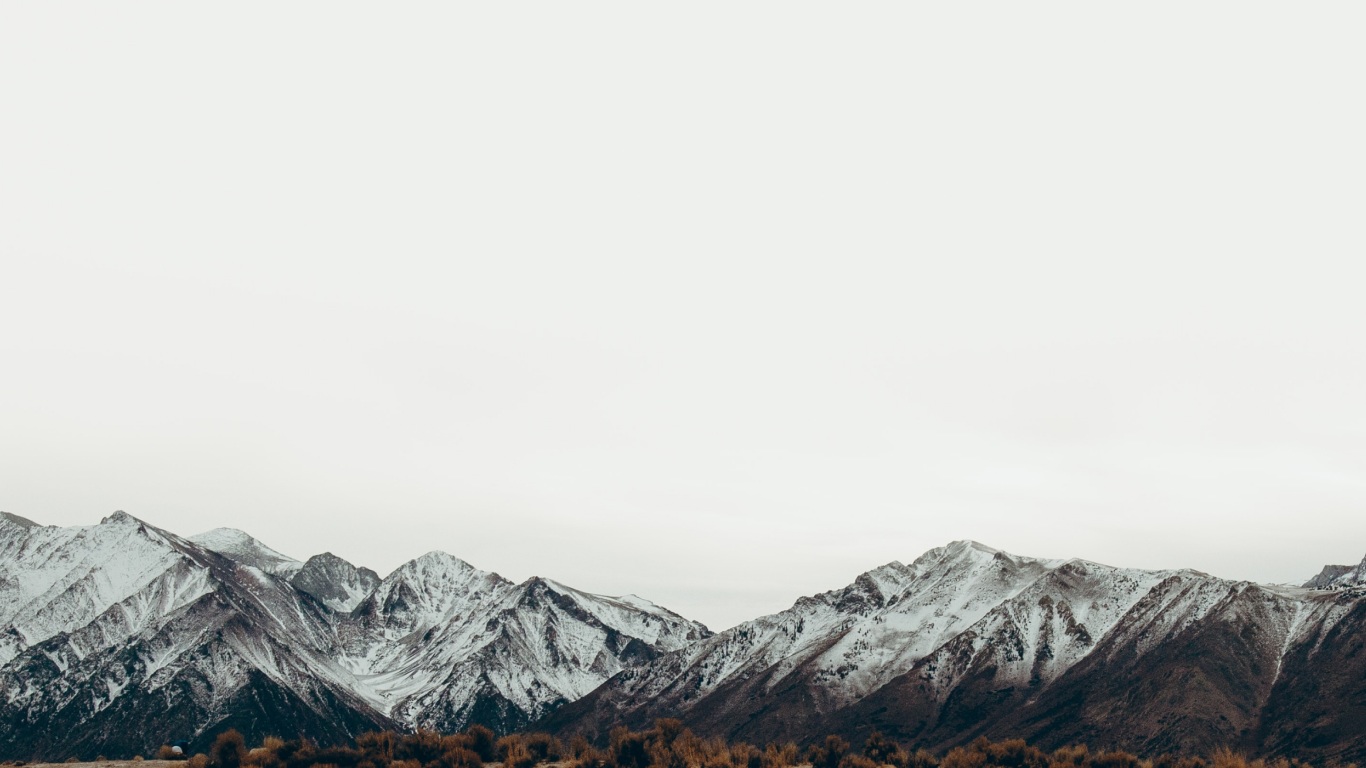
What can data tell us about nature?
We often focus on the way business or society can be understood through the information it produces, but natural and physical science also stand to benefit. In this project, sections of the Roosevelt National Forest were observed to predict the predominant tree cover type from only cartographic (geography related) variables.
An expedition through a project and national forest

Understanding
Cartographic variables include examples such as elevation or hill slope, and are opposed to remotely sensed information like temperature or a satellite image. Given the unique subject matter knowledge in cartography and forest management, a thorough understanding of available data was crucial.

Iterating
No solution to a new problem ever comes from the first try. Instead, learning from previous success and failure can make the most out of attempts in the future. Various data representations, prediction models, and ensemble techniques were evaluated to reach a final solution.

Analyzing
The results of any project must be put into proper context to inform future work and decisions. The relationship of this project to others in natural environments or geography was also assessed for new paths forward.
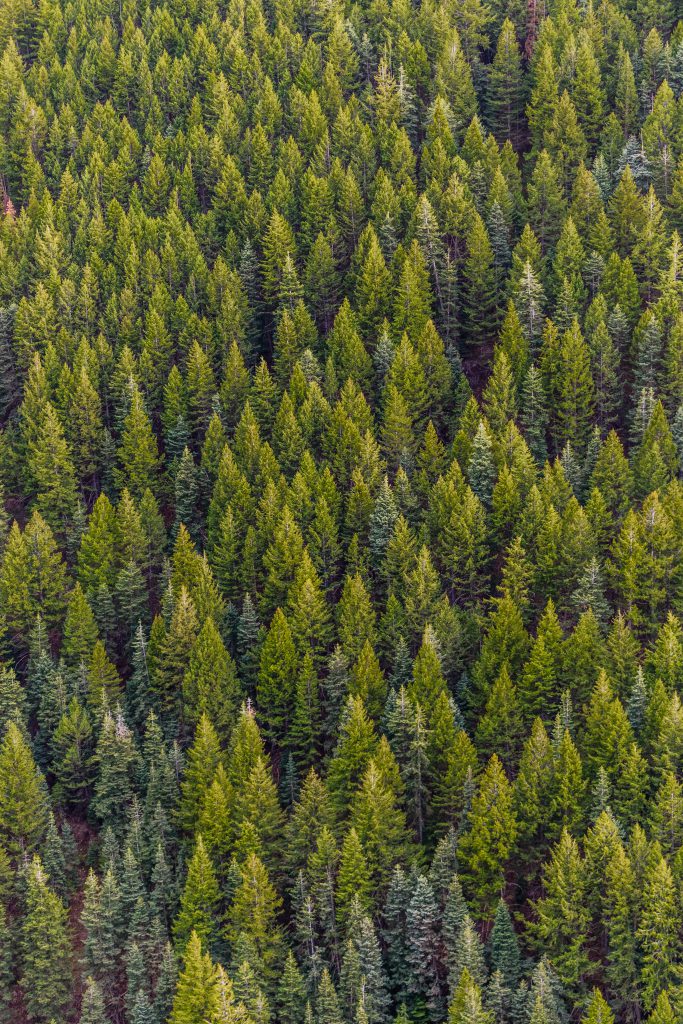
A dataset with more exploration than most
With data from the US Geological Survey and US Forestry Service, there was a variety of subject matter information to become familiar with.
Two is better than one
Similar to other questions where there are minimal constraints on a solution, an ensemble of different models led to more accurate predictions than any single model on its own.
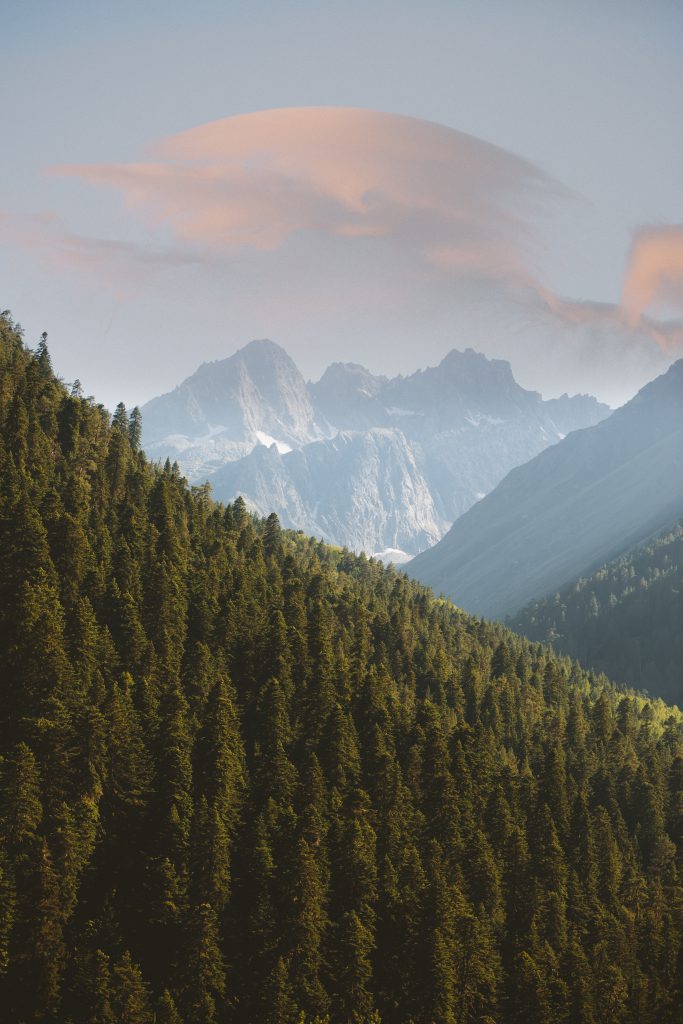
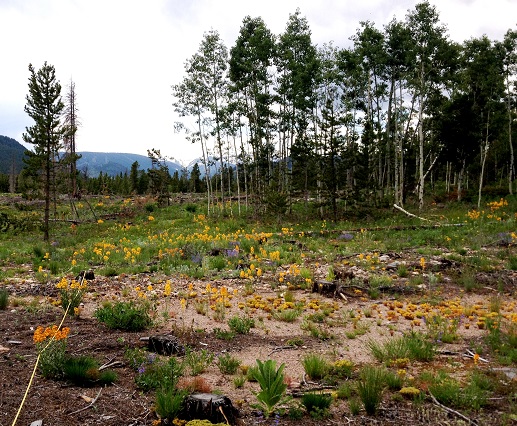
A piece of a unique and important field
Areas not directly commercializable often receive less attention, but that does not affect their value as a scientific endeavor or to society.
Featured Photos
from Roosevelt National Forest, courtesy of US Forest Service
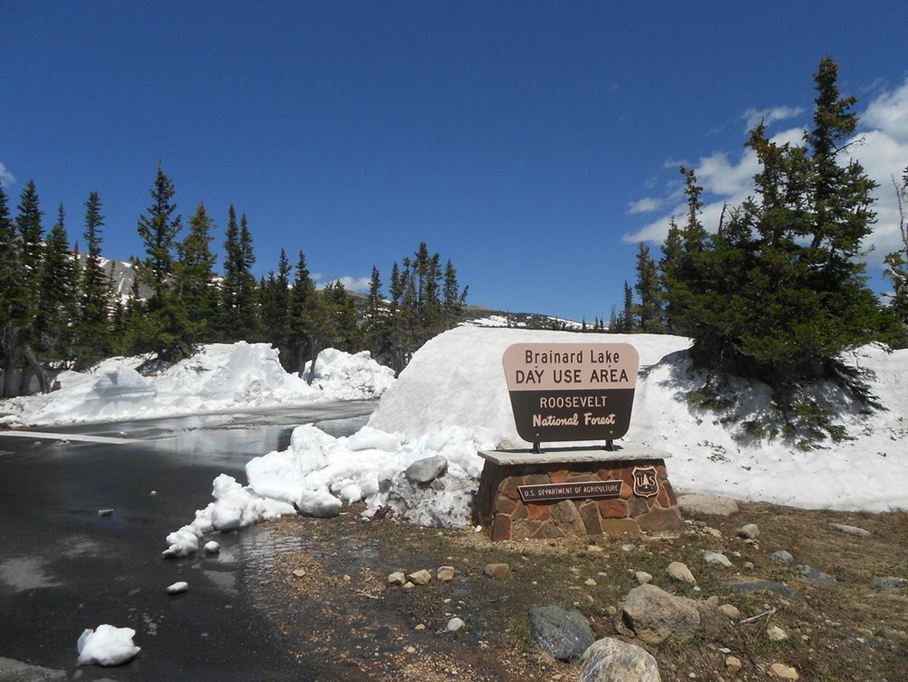
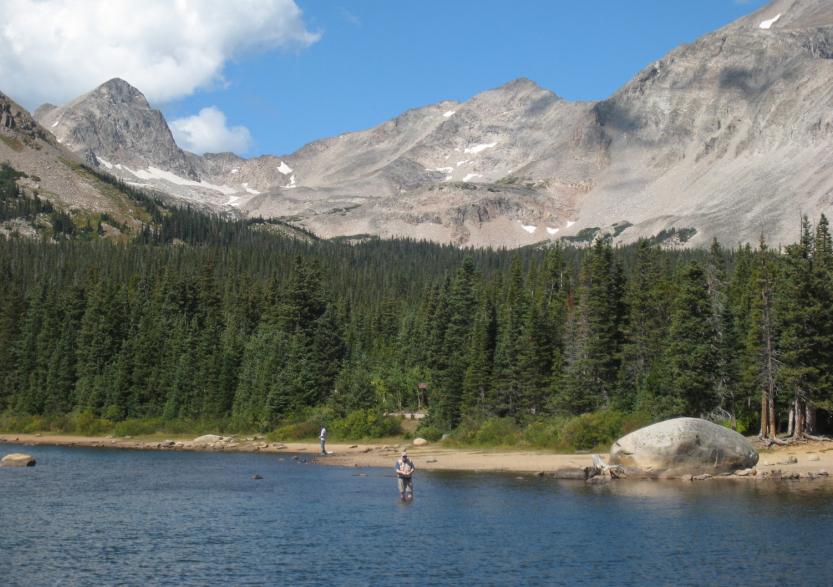
Acknowledgements:
This project was originally hosted as a Kaggle competition in ~2015. After the competition closed, the contributors encountered and designed a submission that serves as the basis for the website.
The original acknowledgements from the Kaggle competition are repeated below:
“Kaggle is hosting this competition for the machine learning community to use for fun and practice. This dataset was provided by Jock A. Blackard and Colorado State University. We also thank the UCI machine learning repository for hosting the dataset. If you use the problem in publication, please cite:
Bache, K. & Lichman, M. (2013). UCI Machine Learning Repository. Irvine, CA: University of California, School of Information and Computer Science“
Contributor & Website Author
Fellow Contributors:
Andi Morey Peterson
Naga Chandrasekaran
Scott Gatzemeier